Mastering AI Implementation Best Practices
Discover the key strategies for a successful AI implementation journey.
4/18/20242 min read
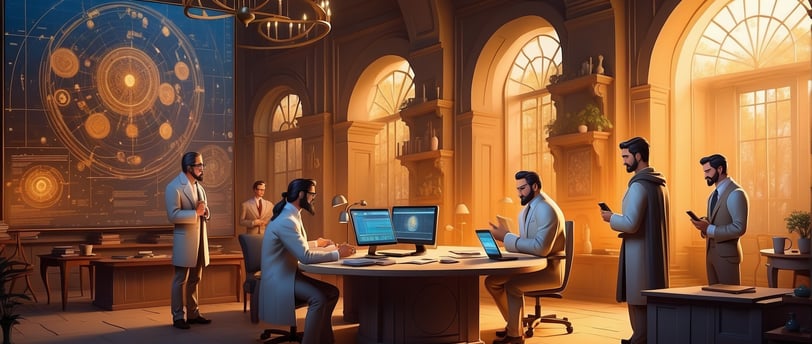
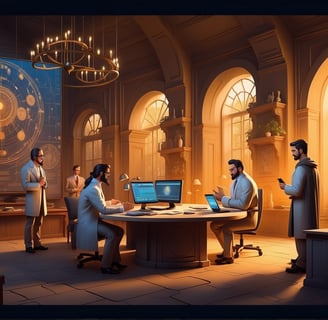
Artificial Intelligence (AI) has become a transformative force across various industries, revolutionizing how businesses operate and innovate. However, implementing AI successfully requires more than just deploying advanced algorithms. It demands a comprehensive understanding of AI implementation best practices to navigate challenges and maximize benefits. In this article, we delve into the strategies and considerations essential for mastering AI implementation.
Importance of AI Implementation Best Practices
Embracing AI implementation best practices is crucial for organizations aiming to unlock the full potential of artificial intelligence. These practices not only enhance operational efficiency but also mitigate potential risks associated with AI adoption. By adhering to best practices, businesses can ensure seamless integration of AI into their processes while safeguarding against pitfalls.
Key Components of AI Implementation Best Practices
Data Quality and Preparation: High-quality data serves as the foundation for successful AI implementation. Organizations must invest in data governance, cleansing, and normalization processes to ensure the reliability and accuracy of input data.
Model Selection and Development: Selecting the appropriate AI models tailored to specific business objectives is paramount. Moreover, continuous refinement and optimization of models through iterative development cycles are essential for maximizing performance.
Ethical Considerations: Ethical implications surrounding AI implementation cannot be overlooked. Organizations must prioritize ethical guidelines and transparency to build trust among stakeholders and mitigate potential biases.
Strategies for Mastering AI Implementation
Cross-functional Collaboration: Effective AI implementation requires collaboration across departments, including IT, data science, legal, and business units. By fostering interdisciplinary teamwork, organizations can leverage diverse expertise and perspectives to drive successful outcomes.
Continuous Learning and Adaptation: AI technologies evolve rapidly, necessitating a culture of continuous learning and adaptation within organizations. Embracing agility and flexibility enables businesses to stay abreast of emerging trends and adapt their AI strategies accordingly.
Challenges in AI Implementation
Data Privacy and Security: Safeguarding sensitive data remains a paramount concern in AI implementation, especially amidst increasing regulatory scrutiny and cybersecurity threats. Organizations must implement robust privacy protocols and encryption mechanisms to protect data integrity.
Bias and Fairness: Addressing bias and fairness issues inherent in AI algorithms is imperative to ensure equitable outcomes. By incorporating fairness metrics and diverse datasets during model development, organizations can mitigate bias and promote inclusivity.
Future Trends in AI Implementation
Advancements in Technology: Continuous advancements in AI technologies, such as deep learning and natural language processing, will drive further innovation in AI implementation. Organizations must embrace emerging technologies to maintain a competitive edge in the evolving landscape.
Regulatory Landscape: Regulatory frameworks governing AI implementation are expected to evolve, necessitating compliance with stringent standards and guidelines. Proactive engagement with regulatory authorities and adherence to ethical principles are essential for navigating regulatory complexities.
Conclusion
Mastering AI implementation best practices is essential for organizations seeking to harness the transformative power of artificial intelligence effectively. By prioritizing data quality, ethical considerations, and cross-functional collaboration, businesses can navigate challenges and unlock new opportunities for innovation and growth in the AI-driven era.
FAQs
Q: How can organizations ensure data quality for AI implementation?
A: Implement robust data governance, conduct thorough cleansing, and leverage automation for validation.
Q: What role does cross-functional collaboration play in AI implementation?
A: Fosters synergy among teams, streamlines decision-making, and ensures alignment with objectives.
Q: What are the key ethical considerations in AI implementation?
A: Transparency, accountability, fairness, and privacy are crucial ethical considerations.
Q: How can businesses address bias in AI algorithms?
A: Diversify datasets, use fairness-aware algorithms, and foster inclusivity within the organization.
Q: What are the future trends in AI implementation strategies?
A: Automation, convergence with other technologies, responsible AI frameworks, and human-centric design.