Mastering Finances with AI Predictions
Learn how AI innovations are optimizing financial forecasting, making smarter investment decisions. Click to explore.
3/22/20243 min read
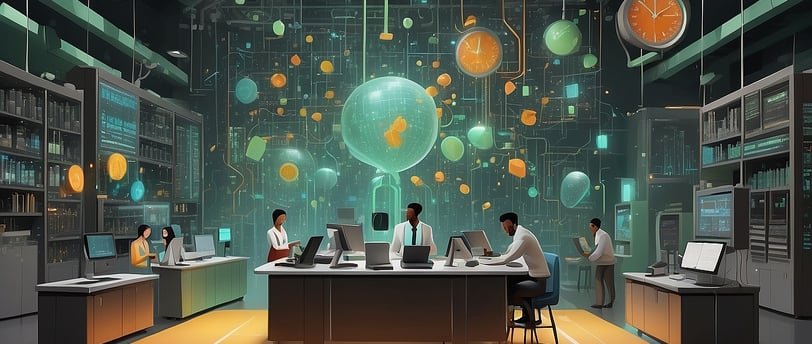
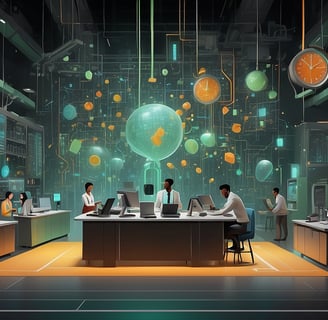
In today's rapidly evolving financial landscape, mastering finances with AI predictions has become a cornerstone for success. With the advent of artificial intelligence (AI) technology, traditional methods of financial analysis and prediction are being revolutionized. This article explores the intricacies of AI predictions in finance, their benefits, challenges, and strategies for effective implementation.
Understanding AI in financial predictions is crucial for navigating the complexities of modern markets. AI algorithms analyze vast amounts of financial data, identify patterns, and make predictions based on historical trends and market indicators. Mastering finances with AI predictions involves leveraging these insights to optimize investment decisions, manage risks, and maximize returns.
How AI Predictions Work in Finance
AI predictions in finance rely on sophisticated algorithms and advanced computational techniques. Data collection involves gathering various financial data points, including market trends, economic indicators, and company performance metrics. Machine learning algorithms process this data, learning from past patterns to make accurate predictions about future market movements. Predictive modeling then generates forecasts, guiding investors and financial professionals in their decision-making processes.
Benefits of Using AI Predictions in Finance
The utilization of AI predictions offers numerous benefits to financial institutions and investors alike. Firstly, AI-driven predictions boast increased accuracy compared to traditional methods, enabling more informed decision-making. Additionally, AI algorithms can analyze vast amounts of data in real-time, providing timely insights into market trends and opportunities. Moreover, AI predictions facilitate risk mitigation by identifying potential threats and helping investors diversify their portfolios accordingly.
Challenges and Limitations
Despite their advantages, AI predictions in finance are not without challenges. Data quality and reliability are paramount concerns, as inaccurate or biased data can skew predictions and lead to suboptimal outcomes. Furthermore, overreliance on algorithms may overlook nuanced market dynamics and human judgment, posing risks in volatile or unforeseen market conditions. Additionally, regulatory concerns surrounding data privacy and algorithmic transparency require careful navigation to ensure compliance and ethical use of AI technology.
Strategies for Mastering Finances with AI Predictions
To effectively master finances with AI predictions, organizations and investors must adopt strategic approaches. This involves integrating AI tools into existing financial systems and workflows, empowering users with actionable insights. Continuous learning and adaptation are essential, as markets evolve and new data becomes available. Moreover, human oversight and intervention are critical for validating AI predictions, mitigating potential errors, and ensuring alignment with strategic objectives.
Successful Implementation of AI in Financial Predictions
Numerous companies have successfully integrated AI into their financial operations, yielding tangible results. For example, investment firms utilize AI-powered algorithms to analyze market trends and optimize trading strategies, resulting in improved returns and reduced risks. Similarly, banks leverage AI predictions for credit risk assessment, streamlining loan approvals and minimizing defaults. These case studies highlight the transformative impact of AI on financial predictions and underscore its potential for driving value creation.
Future Trends in AI Predictions for Finance
Looking ahead, the future of AI predictions in finance is marked by continued advancements in technology and evolving market dynamics. AI algorithms will become increasingly sophisticated, capable of processing unstructured data sources such as social media sentiment and news articles. Ethical considerations surrounding AI use will gain prominence, driving regulatory frameworks and industry standards. Moreover, the democratization of AI technology will expand access to predictive analytics, empowering individuals and businesses to make informed financial decisions.
Conclusion
In conclusion, mastering finances with AI predictions is essential for navigating today's complex financial landscape. By harnessing the power of AI technology, investors and financial professionals can gain valuable insights, optimize decision-making, and mitigate risks. However, challenges such as data quality and regulatory compliance must be addressed to unlock the full potential of AI predictions in finance. As technology continues to evolve, AI will play an increasingly integral role in shaping the future of finance, driving innovation and driving value creation.
FAQs (Frequently Asked Questions)
Q) What types of data are used in AI predictions for finance?
A: AI predictions in finance utilize various data sources, including market trends, economic indicators, company financials, and consumer behavior.
Q: How accurate are AI predictions compared to traditional forecasting methods?
A: AI predictions often boast higher accuracy rates than traditional methods, thanks to advanced algorithms and real-time data analysis.
Q: Are there any ethical concerns associated with AI predictions in finance?
A: Ethical concerns surrounding AI predictions include data privacy, algorithmic bias, and transparency in decision-making processes.
Q: Can AI predictions replace human judgment in financial decision-making?
A: While AI predictions offer valuable insights, human judgment remains crucial for validating predictions, interpreting results, and navigating unforeseen market conditions.
Q: What are some future trends in AI predictions for finance?
A: Future trends in AI predictions for finance include advancements in technology, increased regulatory scrutiny, and greater accessibility to predictive analytics tools.